BCI Kickstarter #03: EEG Signal Acquisition and Processing
Welcome back to our BCI crash course! In the previous blog, we explored the basic concepts of BCIs and delved into the fundamentals of neuroscience. Now, it's time to get our hands dirty with the practical aspects of EEG signal acquisition and processing. This blog will guide you through the journey of transforming raw EEG data into a format suitable for meaningful analysis and BCI applications. We will cover signal preprocessing techniques, and feature extraction methods, providing you with the essential tools for decoding the brain's electrical secrets.
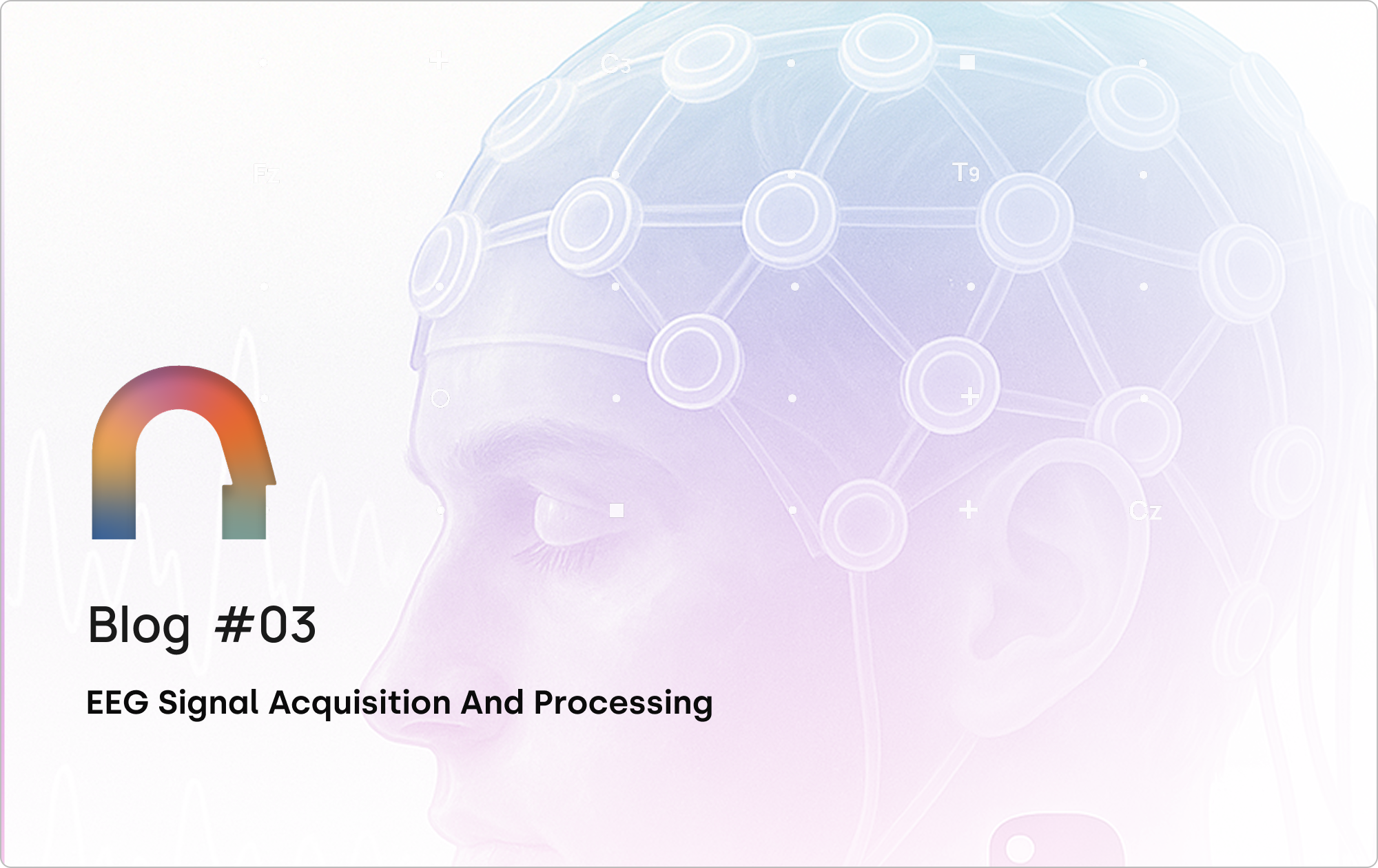
Signal Preprocessing Techniques: Cleaning Up the Data
Raw EEG data, fresh from the electrodes, is often a noisy and complex landscape. To extract meaningful insights and develop reliable BCIs, we need to apply various signal preprocessing techniques to clean up the data, remove artifacts, and enhance the true brain signals.
Why Preprocessing is Necessary: Navigating a Sea of Noise
The journey from raw EEG recordings to usable data is fraught with challenges:
- Noise and Artifacts Contamination: EEG signals are susceptible to various sources of interference, both biological (e.g., muscle activity, eye blinks, heartbeats) and environmental (e.g., power line noise, electrode movement). These artifacts can obscure the true brain signals we are interested in.
- Separating True Brain Signals: Even in the absence of obvious artifacts, raw EEG data contains a mix of neural activity related to various cognitive processes. Preprocessing helps us isolate the specific signals relevant to our research or BCI application.
Importing Data: Laying the Foundation
Before we can begin preprocessing, we need to import our EEG data into a suitable software environment. Common EEG data formats include:
- FIF (Functional Imaging File Format): A widely used format developed for MEG and EEG data, supported by the MNE library in Python.
- EDF (European Data Format): Another standard format, often used for clinical EEG recordings.
Libraries like MNE provide functions for reading and manipulating these formats, enabling us to work with EEG data in a programmatic way.
Removing Bad Channels and Interpolation: Dealing with Faulty Sensors
Sometimes, EEG recordings contain bad channels — electrodes that are malfunctioning, poorly placed, or picking up excessive noise. We need to identify and address these bad channels before proceeding with further analysis.
Identifying Bad Channels:
- Visual Inspection: Plotting the raw EEG data and visually identifying channels with unusually high noise levels, flat lines, or other anomalies.
- Automated Methods: Using algorithms that detect statistically significant deviations from expected signal characteristics.
Interpolation:
If a bad channel cannot be salvaged, we can use interpolation to estimate its missing data based on the surrounding good channels. Spherical spline interpolation is a common technique that projects electrode locations onto a sphere and uses a mathematical model to estimate the missing values.
Filtering: Tuning into the Right Frequencies
Filtering is a fundamental preprocessing step that allows us to remove unwanted frequencies from our EEG signal. Different types of filters serve distinct purposes:
- High-Pass Filtering: Removes slow drifts and DC offsets, which are often caused by electrode movement or skin potentials. A typical cutoff frequency for high-pass filtering is around 0.1 Hz.
- Low-Pass Filtering: Removes high-frequency noise, which can originate from muscle activity or electrical interference. A common cutoff frequency for low-pass filtering is around 30 Hz for most cognitive tasks, though some applications may use higher cutoffs for studying gamma activity.
- Band-Pass Filtering: Combines high-pass and low-pass filtering to isolate a specific frequency band of interest, such as the alpha (8-12 Hz) or beta (12-30 Hz) band.
- Notch Filtering: Removes a narrow band of frequencies, typically used to eliminate power line noise (50/60 Hz) or other specific interference.
Choosing the appropriate filter settings is crucial for isolating the relevant brain signals and minimizing the impact of noise on our analysis.
Downsampling: Reducing the Data Load
Downsampling refers to reducing the sampling rate of our EEG signal, which can be beneficial for:
- Reducing data storage requirements: Lower sampling rates result in smaller file sizes.
- Improving computational efficiency: Processing lower-resolution data requires less computing power.
However, we need to be cautious when downsampling to avoid losing important information. The Nyquist-Shannon sampling theorem dictates that we must sample at a rate at least twice the highest frequency of interest in our signal to avoid aliasing, where high frequencies are incorrectly represented as lower frequencies.
Decimation is a common downsampling technique that combines low-pass filtering with sample rate reduction to ensure that we don't introduce aliasing artifacts into our data.
Re-Referencing: Choosing Your Point of View
In EEG recording, each electrode's voltage is measured relative to a reference electrode. The choice of reference can significantly influence the interpretation of our signals, as it affects the baseline against which brain activity is measured.
Common reference choices include:
- Linked Mastoids: Averaging the signals from the mastoid electrodes behind each ear.
- Average Reference: Averaging the signals from all electrodes.
- Other References: Specific electrodes (e.g., Cz) or combinations of electrodes can be chosen based on the research question or BCI application.
Re-referencing allows us to change the reference of our EEG data after it's been recorded. This can be useful for comparing data recorded with different reference schemes or for exploring the impact of different references on signal interpretation. Libraries like MNE provide functions for easily re-referencing data.
Feature Extraction Methods: Finding the Signal in the Noise
Once we've preprocessed our EEG data, it's time to extract meaningful information that can be used for analysis or to train BCI systems. Feature extraction is the process of transforming the preprocessed EEG signal into a set of representative features that capture the essential patterns and characteristics of the underlying brain activity.
What is Feature Extraction? Simplifying the Data Landscape
Raw EEG data, even after preprocessing, is often high-dimensional and complex. Feature extraction serves several important purposes:
- Reducing Data Dimensionality: By extracting a smaller set of representative features, we simplify the data, making it more manageable for analysis and machine learning algorithms.
- Highlighting Relevant Patterns: Feature extraction methods focus on specific aspects of the EEG signal that are most relevant to the research question or BCI application, enhancing the signal-to-noise ratio and improving the accuracy of our analyses.
Time-Domain Features: Analyzing Signal Fluctuations
Time-domain features capture the temporal characteristics of the EEG signal, focusing on how the voltage changes over time. Some common time-domain features include:
- Amplitude:
- Peak-to-Peak Amplitude: The difference between the highest and lowest voltage values within a specific time window.
- Mean Amplitude: The average voltage value over a given time period.
- Variance: A measure of how much the signal fluctuates around its mean value.
- Peak-to-Peak Amplitude: The difference between the highest and lowest voltage values within a specific time window.
- Latency:
- Onset Latency: The time it takes for a specific event-related potential (ERP) component to appear after a stimulus.
- Peak Latency: The time point at which an ERP component reaches its maximum amplitude.
- Onset Latency: The time it takes for a specific event-related potential (ERP) component to appear after a stimulus.
- Time-Series Analysis:
- Autoregressive Models: Statistical models that predict future values of the signal based on its past values, capturing temporal dependencies in the data.
- Moving Averages: Smoothing techniques that calculate the average of the signal over a sliding window, reducing noise and highlighting trends.
- Autoregressive Models: Statistical models that predict future values of the signal based on its past values, capturing temporal dependencies in the data.
Frequency-Domain Features: Unveiling the Brain's Rhythms
Frequency-domain features analyze the EEG signal in the frequency domain, revealing the power distribution across different frequency bands. Key frequency-domain features include:
- Power Spectral Density (PSD): A measure of the signal's power at different frequencies. PSD is typically calculated using the Fast Fourier Transform (FFT), which decomposes the signal into its constituent frequencies.
- Band Power: The total power within a specific frequency band, such as delta, theta, alpha, beta, or gamma. Band power features are often used in BCI systems to decode mental states or user intent.
Time-Frequency Features: Bridging the Time and Frequency Divide
Time-frequency features provide a combined view of the EEG signal in both time and frequency domains, capturing dynamic changes in frequency content over time. Important time-frequency features include:
- Wavelet Transform: A powerful technique that decomposes the signal into a set of wavelets, functions that vary in both frequency and time duration. Wavelet transforms excel at capturing transient events and analyzing signals with non-stationary frequency content.
- Short-Time Fourier Transform (STFT): Divides the signal into short segments and calculates the FFT for each segment, providing a time-varying spectrum. STFT is useful for analyzing how the frequency content of the signal changes over time.
From Raw Signals to Actionable Insights
The journey from raw EEG data to meaningful insights and BCI control involves a carefully orchestrated sequence of signal acquisition, preprocessing, and feature extraction. Each step plays a crucial role in revealing the hidden patterns within the brain's electrical symphony, allowing us to decode mental states, control external devices, and unlock new possibilities for human-computer interaction.
By mastering these techniques, we can transform the complex and noisy world of EEG recordings into a rich source of information, paving the way for innovative BCI applications that can improve lives and expand our understanding of the human brain.
Further Reading and Resources
- Book: Analyzing Neural Time Series Data: Theory and Practice
By: Mike X Cohen
https://doi.org/10.7551/mitpress/9609.001.0001
ISBN (electronic): 9780262319553
- Tutorial: MNE-Python documentation on preprocessing: https://mne.tools/stable/auto_tutorials/preprocessing/index.html
- Article: Urigüen, J. A., & Garcia-Zapirain, B. (2015). EEG artifact removal—state-of-the-art and guidelines. Journal of Neural Engineering, 12(3), 031001.
What's Next: Real-World BCIs using Signal Processing
This concludes our exploration of EEG signal acquisition and processing. Now that we've learned how to clean up and extract meaningful features from raw EEG data, we are ready to explore how these techniques are used to build real-world BCI applications.
In the next post, we'll dive into the fascinating world of BCI paradigms and applications, discovering the diverse ways BCIs are being used to translate brain signals into actions. Stay tuned!

In our previous blog, we explored how biosignals serve as the body's internal language—electrical, mechanical, and chemical messages that allow us to understand and interface with our physiology. Among these, electrical biosignals are particularly important for understanding how our nervous system, muscles, and heart function in real time. In this article, we’ll take a closer look at three of the most widely used electrical biosignals—EEG, ECG, and EMG—and their growing role in neurotechnology, diagnostics, performance tracking, and human-computer interaction. If you're new to the concept of biosignals, you might want to check out our introductory blog for a foundational overview.
"The body is a machine, and we must understand its currents if we are to understand its functions."-Émil du Bois-Reymond, pioneer in electrophysiology.
Life, though rare in the universe, leaves behind unmistakable footprints—biosignals. These signals not only confirm the presence of life but also narrate what a living being is doing, feeling, or thinking. As technology advances, we are learning to listen to these whispers of biology. Whether it’s improving health, enhancing performance, or building Brain-Computer Interfaces (BCIs), understanding biosignals is key.
Among the most studied biosignals are:
- Electroencephalogram (EEG) – from the brain
- Electrocardiogram (ECG) – from the heart
- Electromyogram (EMG) – from muscles
- Galvanic Skin Response (GSR) – from skin conductance
These signals are foundational for biosignal processing, real-time monitoring, and interfacing the human body with machines. In this article we look at some of these biosignals and some fascinating stories behind them.
Electroencephalography (EEG): Listening to Brainwaves
In 1893, a 19 year old Hans Berger fell from a horse and had a near death experience. Little did he know that it would be a pivotal moment in the history of neurotechnology. The same day he received a telegram from his sister who was extremely concerned for him because she had a bad feeling. Hans Berger was convinced that this was due to the phenomenon of telepathy. After all, it was the age of radio waves, so why can’t there be “brain waves”? In his ensuing 30 year career telepathy was not established but in his pursuit, Berger became the first person to record brain waves.
When neurons fire together, they generate tiny electrical currents. These can be recorded using electrodes placed on the scalp (EEG), inside the skull (intracranial EEG), or directly on the brain (ElectroCorticogram). EEG signal processing is used not only to understand the brain’s rhythms but also in EEG-based BCI systems, allowing communication and control for people with paralysis. Event-Related Potentials (ERPs) and Local Field Potentials (LFPs) are specialized types of EEG signals that provide insights into how the brain responds to specific stimuli.

Electrocardiogram (ECG): The Rhythm of the Heart
The heart has its own internal clock which produces tiny electrical signals every time it beats. Each heartbeat starts with a small electrical impulse made by a special part of the heart called the sinoatrial (SA) node. This impulse spreads through the heart muscle and makes it contract, first the upper (atria) and then lower chambers (ventricles) – that’s what pumps blood. This process produces voltage changes, which can be recorded via electrodes on the skin.
This gives rise to the classic PQRST waveform, with each component representing a specific part of the heart’s cycle. Modern wearables and medical devices use ECG signal analysis to monitor heart health in real time.
Fun fact: The waveform starts with “P” because Willem Einthoven left room for earlier letters—just in case future scientists discovered pre-P waves! So, thanks to a cautious scientist, we have the quirky naming system we still follow today.

Electromyography (EMG): The Language of Movement
When we perform any kind of movement - lifting our arm, kicking our leg, smiling, blinking or even breathing- our brain sends electrical signals to our muscles telling them to contract. When these neurons, known as motor neurons fire they release electrical impulses that travel to the muscle, causing it to contract. This electrical impulse—called a motor unit action potential (MUAP)—is what we see as an EMG signal. So, every time we move, we are generating an EMG signal!

Medical Applications
Medically, EMG is used for monitoring muscle fatigue especially in rehabilitation settings and muscle recovery post-injury or surgery. This helps clinicians measure progress and optimize therapy. EMG can distinguish between voluntary and involuntary movements, making it useful in diagnosing neuromuscular disorders, assessing stroke recovery, spinal cord injuries, and motor control dysfunctions.
Performance and Sports Science
In sports science, EMG can tell us muscle-activation timing and quantify force output of muscle groups. These are important factors to measure performance improvement in any sport. The number of motor units recruited and the synergy between muscle groups, helps us capture “mind-muscle connection” and muscle memory. Such things which were previously spoken off in a figurative manner can be scientifically measured and quantified using EMG. By tracking these parameters we get a window into movement efficiency and athletic performance. EMG is also used for biofeedback training, enabling individuals to consciously correct poor movement habits or retrain specific muscles
Beyond medicine and sports, EMG is used for gesture recognition in AR/VR and gaming, silent speech detection via facial EMG, and next-gen prosthetics and wearable exosuits that respond to the user’s muscle signals. EMG can be used in brain-computer interfaces (BCIs), helping paralyzed individuals control digital devices or communicate through subtle muscle activity. EMG bridges the gap between physiology, behavior, and technology—making it a critical tool in healthcare, performance optimization, and human-machine interaction.
As biosignal processing becomes more refined and neurotech devices more accessible, we are moving toward a world where our body speaks—and machines understand. Whether it’s detecting the subtlest brainwaves, tracking a racing heart, or interpreting muscle commands, biosignals are becoming the foundation of the next digital revolution. One where technology doesn’t just respond, but understands.

The human body is constantly generating data—electrical impulses, chemical fluctuations, and mechanical movements—that provide deep insights into our bodily functions, and cognitive states. These measurable physiological signals, known as biosignals, serve as the body's natural language, allowing us to interpret and interact with its inner workings. From monitoring brain activity to assessing muscle movement, biosignals are fundamental to understanding human physiology and expanding the frontiers of human-machine interaction. But what exactly are biosignals? How are they classified, and why do they matter? In this blog, we will explore the different types of biosignals, the science behind their measurement, and the role they play in shaping the future of human health and technology.
What are Biosignals?
Biosignals refer to any measurable signal originating from a biological system. These signals are captured and analyzed to provide meaningful information about the body's functions. Traditionally used in medicine for diagnosis and monitoring, biosignals are now at the forefront of research in neurotechnology, wearable health devices, and human augmentation.
The Evolution of Biosignal Analysis
For centuries, physicians have relied on pulse measurements to assess a person’s health. In ancient Chinese and Ayurvedic medicine, the rhythm, strength, and quality of the pulse were considered indicators of overall well-being. These early methods, while rudimentary, laid the foundation for modern biosignal monitoring.
Today, advancements in sensor technology, artificial intelligence, and data analytics have transformed biosignal analysis. Wearable devices can continuously track heart rate, brain activity, and oxygen levels with high precision. AI-driven algorithms can detect abnormalities in EEG or ECG signals, helping diagnose neurological and cardiac conditions faster than ever. Real-time biosignal monitoring is now integrated into medical, fitness, and neurotechnology applications, unlocking insights that were once beyond our reach.
This leap from manual pulse assessments to AI-powered biosensing is reshaping how we understand and interact with our own biology.
Types of Biosignals:-
Biosignals come in three main types
- Electrical Signals: Electrical signals are generated by neural and muscular activity, forming the foundation of many biosignal applications. Electroencephalography (EEG) captures brain activity, playing a crucial role in understanding cognition and diagnosing neurological disorders. Electromyography (EMG) measures muscle activity, aiding in rehabilitation and prosthetic control. Electrocardiography (ECG) records heart activity, making it indispensable for cardiovascular monitoring. Electrooculography (EOG) tracks eye movements, often used in vision research and fatigue detection.
- Mechanical Signals: Mechanical signals arise from bodily movements and structural changes, providing valuable physiological insights. Respiration rate tracks breathing patterns, essential for sleep studies and respiratory health. Blood pressure serves as a key indicator of cardiovascular health and stress responses. Muscle contractions help in analyzing movement disorders and biomechanics, enabling advancements in fields like sports science and physical therapy.
- Chemical Signals: Chemical signals reflect the biochemical activity within the body, offering a deeper understanding of physiological states. Neurotransmitters like dopamine and serotonin play a critical role in mood regulation and cognitive function. Hormone levels serve as indicators of stress, metabolism, and endocrine health. Blood oxygen levels are vital for assessing lung function and metabolic efficiency, frequently monitored in medical and athletic settings.
How Are Biosignals Measured?
After understanding what biosignals are and their different types, the next step is to explore how these signals are captured and analyzed. Measuring biosignals requires specialized sensors that detect physiological activity and convert it into interpretable data. This process involves signal acquisition, processing, and interpretation, enabling real-time monitoring and long-term health assessments.
- Electrodes & Wearable Sensors
Electrodes measure electrical biosignals like EEG (brain activity), ECG (heart activity), and EMG (muscle movement) by detecting small voltage changes. Wearable sensors, such as smartwatches, integrate these electrodes for continuous, non-invasive monitoring, making real-time health tracking widely accessible.
- Optical Sensors
Optical sensors, like pulse oximeters, use light absorption to measure blood oxygen levels (SpO₂) and assess cardiovascular and respiratory function. They are widely used in fitness tracking, sleep studies, and medical diagnostics.
- Pressure Sensors
These sensors measure mechanical biosignals such as blood pressure, respiratory rate, and muscle contractions by detecting force or air pressure changes. Blood pressure cuffs and smart textiles with micro-pressure sensors provide valuable real-time health data.
- Biochemical Assays
Biochemical sensors detect chemical biosignals like hormones, neurotransmitters, and metabolic markers. Advanced non-invasive biosensors can now analyze sweat composition, hydration levels, and electrolyte imbalances without requiring a blood sample.
- Advanced AI & Machine Learning in Biosignal Analysis
Artificial intelligence (AI) and machine learning (ML) have transformed biosignal interpretation by enhancing accuracy and efficiency. These technologies can detect abnormalities in EEG, ECG, and EMG signals, helping with early disease diagnosis. They also filter out noise and artifacts, improving signal clarity for more precise analysis. By analyzing long-term biosignal trends, AI can predict potential health risks and enable proactive interventions. Additionally, real-time AI-driven feedback is revolutionizing applications like neurofeedback and biofeedback therapy, allowing for more personalized and adaptive healthcare solutions. The integration of AI with biosignal measurement is paving the way for smarter diagnostics, personalized medicine, and enhanced human performance tracking.
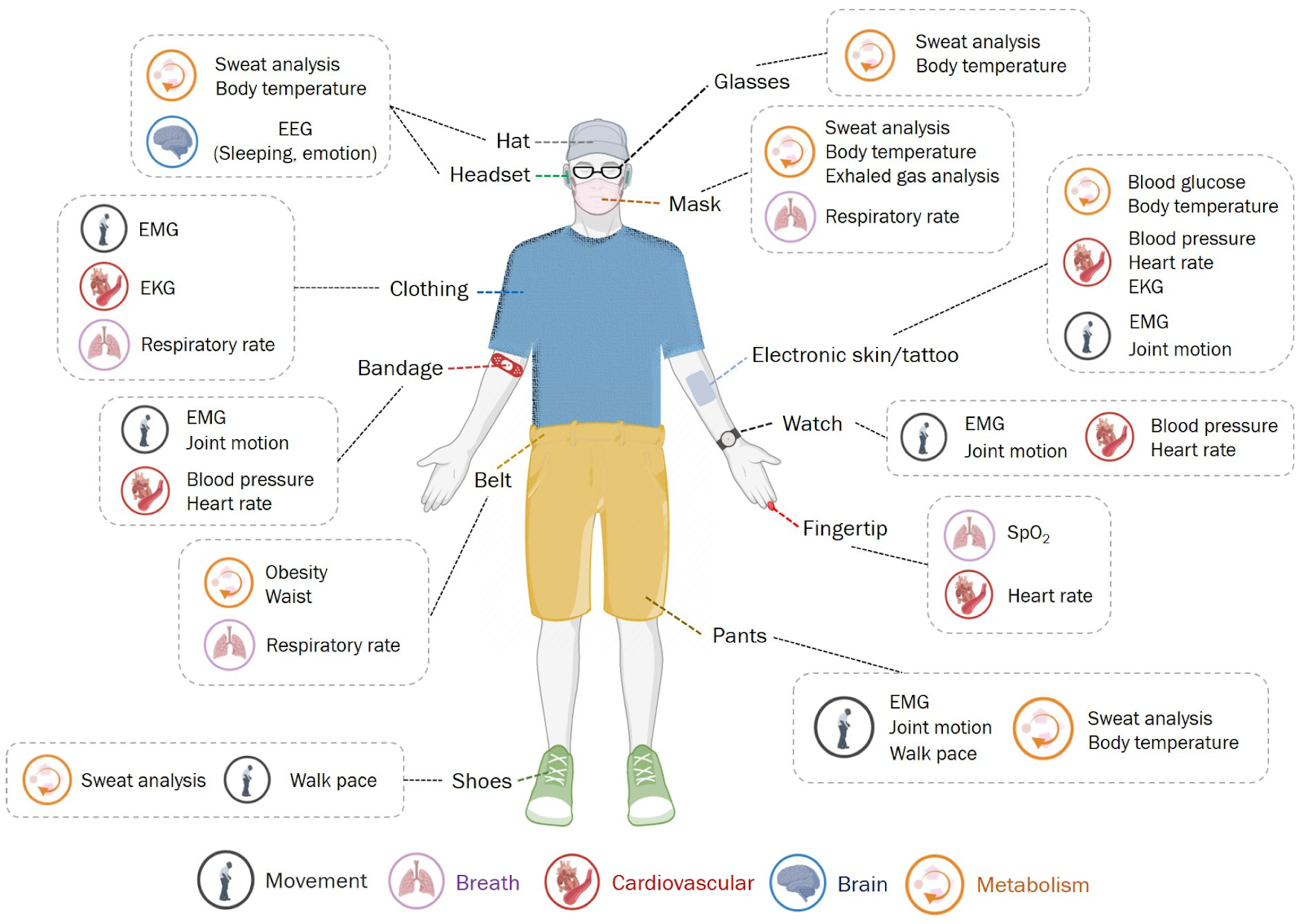
Figure : The image provides an overview of biosignals detectable from different parts of the human body and their corresponding wearable sensors. It categorizes biosignals such as EEG, ECG, and EMG, demonstrating how wearable technologies enable real-time health monitoring and improve diagnostic capabilities.
The Future of Biosignals
As sensor technology and artificial intelligence continue to evolve, biosignals will become even more integrated into daily life, shifting from reactive healthcare to proactive and predictive wellness solutions. Advances in non-invasive monitoring will allow for continuous tracking of vital biomarkers, reducing the need for clinical testing. Wearable biosensors will provide real-time insights into hydration, stress, and metabolic health, enabling individuals to make data-driven decisions about their well-being. Artificial intelligence will play a pivotal role in analyzing complex biosignal patterns, enabling early detection of diseases before symptoms arise and personalizing treatments based on an individual's physiological data.
The intersection of biosignals and brain-computer interfaces (BCIs) is also pushing the boundaries of human-machine interaction. EEG-based BCIs are already enabling users to control digital interfaces with their thoughts, and future developments could lead to seamless integration between the brain and external devices. Beyond healthcare, biosignals will drive innovations in adaptive learning, biometric authentication, and even entertainment, where music, lighting, and virtual experiences could respond to real-time physiological states. As these technologies advance, biosignals will not only help us understand the body better but also enhance human capabilities, bridging the gap between biology and technology in unprecedented ways.

Welcome back to our BCI crash course! Over the past eight blogs, we have explored the fascinating intersection of neuroscience, engineering, and machine learning, from the fundamental concepts of BCIs to the practical implementation of real-world applications. In this final installment, we will shift our focus to the future of BCI, delving into advanced topics and research directions that are pushing the boundaries of mind-controlled technology. Get ready to explore the exciting possibilities of hybrid BCIs, adaptive algorithms, ethical considerations, and the transformative potential that lies ahead for this groundbreaking field.
Hybrid BCIs: Combining Paradigms for Enhanced Performance
As we've explored in previous posts, different BCI paradigms leverage distinct brain signals and have their strengths and limitations. Motor imagery BCIs excel at decoding movement intentions, P300 spellers enable communication through attention-based selections, and SSVEP BCIs offer high-speed control using visual stimuli.
What are Hybrid BCIs? Synergy of Brain Signals
Hybrid BCIs combine multiple BCI paradigms, integrating different brain signals to create more robust, versatile, and user-friendly systems. Imagine a BCI that leverages both motor imagery and SSVEP to control a robotic arm with greater precision and flexibility, or a system that combines P300 with error-related potentials (ErrPs) to improve the accuracy and speed of a speller.
Benefits of Hybrid BCIs: Unlocking New Possibilities
Hybrid BCIs offer several advantages over single-paradigm systems:
- Improved Accuracy and Reliability: Combining complementary brain signals can enhance the signal-to-noise ratio and reduce the impact of individual variations in brain activity, leading to more accurate and reliable BCI control.
- Increased Flexibility and Adaptability: Hybrid BCIs can adapt to different user needs, tasks, and environments by dynamically switching between paradigms or combining them in a way that optimizes performance.
- Richer and More Natural Interactions: Integrating multiple BCI paradigms opens up possibilities for creating more intuitive and natural BCI interactions, allowing users to control devices with a greater range of mental commands.
Examples of Hybrid BCIs: Innovations in Action
Research is exploring various hybrid BCI approaches:
- Motor Imagery + SSVEP: Combining motor imagery with SSVEP can enhance the control of robotic arms. Motor imagery provides continuous control signals for movement direction, while SSVEP enables discrete selections for grasping or releasing objects.
- P300 + ErrP: Integrating P300 with ErrPs, brain signals that occur when we make errors, can improve speller accuracy. The P300 is used to select letters, while ErrPs can be used to automatically correct errors, reducing the need for manual backspacing.
Adaptive BCIs: Learning and Evolving with the User
One of the biggest challenges in BCI development is the inherent variability in brain signals. A BCI system that works perfectly for one user might perform poorly for another, and even a single user's brain activity can change over time due to factors like learning, fatigue, or changes in attention. This is where adaptive BCIs come into play, offering a dynamic and personalized approach to brain-computer interaction.
The Need for Adaptation: Embracing the Brain's Dynamic Nature
BCI systems need to adapt to several factors:
- Changes in User Brain Activity: Brain signals are not static. They evolve as users learn to control the BCI, become fatigued, or shift their attention. An adaptive BCI can track these changes and adjust its processing accordingly.
- Variations in Signal Quality and Noise: EEG recordings can be affected by various sources of noise, from muscle artifacts to environmental interference. An adaptive BCI can adjust its filtering and artifact rejection parameters to maintain optimal signal quality.
- Different User Preferences and Skill Levels: BCI users have different preferences for control strategies, feedback modalities, and interaction speeds. An adaptive BCI can personalize its settings to match each user's individual needs and skill level.
Methods for Adaptation: Tailoring BCIs to the Individual
Various techniques can be employed to create adaptive BCIs:
- Machine Learning Adaptation: Machine learning algorithms, such as those used for classification, can be trained to continuously learn and update the BCI model based on the user's brain data. This allows the BCI to adapt to changes in brain patterns over time and improve its accuracy and responsiveness.
- User Feedback Adaptation: BCIs can incorporate user feedback, either explicitly (through direct input) or implicitly (by monitoring performance and user behavior), to adjust parameters and optimize the interaction. For example, if a user consistently struggles to control a motor imagery BCI, the system could adjust the classification thresholds or provide more frequent feedback to assist them.
Benefits of Adaptive BCIs: A Personalized and Evolving Experience
Adaptive BCIs offer significant advantages:
- Enhanced Usability and User Experience: By adapting to individual needs and preferences, adaptive BCIs can become more intuitive and easier to use, reducing user frustration and improving the overall experience.
- Improved Long-Term Performance and Reliability: Adaptive BCIs can maintain high levels of performance and reliability over time by adjusting to changes in brain activity and signal quality.
- Personalized BCIs: Adaptive algorithms can tailor the BCI to each user's unique brain patterns, preferences, and abilities, creating a truly personalized experience.
Ethical Considerations: Navigating the Responsible Development of BCI
As BCI technology advances, it's crucial to consider the ethical implications of its development and use. BCIs have the potential to profoundly impact individuals and society, raising questions about privacy, autonomy, fairness, and responsibility.
Introduction: Ethics at the Forefront of BCI Innovation
Ethical considerations should be woven into the fabric of BCI research and development, guiding our decisions and ensuring that this powerful technology is used for good.
Key Ethical Concerns: Navigating a Complex Landscape
- Privacy and Data Security: BCIs collect sensitive brain data, raising concerns about privacy violations and potential misuse. Robust data security measures and clear ethical guidelines are crucial for protecting user privacy and ensuring responsible data handling.
- Agency and Autonomy: BCIs have the potential to influence user thoughts, emotions, and actions. It's essential to ensure that BCI use respects user autonomy and agency, avoiding coercion, manipulation, or unintended consequences.
- Bias and Fairness: BCI algorithms can inherit biases from the data they are trained on, potentially leading to unfair or discriminatory outcomes. Addressing these biases and developing fair and equitable BCI systems is essential for responsible innovation.
- Safety and Responsibility: As BCIs become more sophisticated and integrated into critical applications like healthcare and transportation, ensuring their safety and reliability is paramount. Clear lines of responsibility and accountability need to be established to mitigate potential risks and ensure ethical use.
Guidelines and Principles: A Framework for Responsible BCI
Efforts are underway to establish ethical guidelines and principles for BCI research and development. These guidelines aim to promote responsible innovation, protect user rights, and ensure that BCI technology benefits society as a whole.
Current Challenges and Future Prospects: The Road Ahead for BCI
While BCI technology has made remarkable progress, several challenges remain to be addressed before it can fully realize its transformative potential. However, the future of BCI is bright, with exciting possibilities on the horizon for enhancing human capabilities, restoring lost function, and improving lives.
Technical Challenges: Overcoming Roadblocks to Progress
- Signal Quality and Noise: Non-invasive BCIs, particularly those based on EEG, often suffer from low signal-to-noise ratios. Improving signal quality through advanced electrode designs, noise reduction algorithms, and a better understanding of brain signals is crucial for enhancing BCI accuracy and reliability.
- Robustness and Generalizability: Current BCI systems often work well in controlled laboratory settings but struggle to perform consistently across different users, environments, and tasks. Developing more robust and generalizable BCIs is essential for wider adoption and real-world applications.
- Long-Term Stability: Maintaining the long-term stability and performance of BCI systems, especially for implanted devices, is a significant challenge. Addressing issues like biocompatibility, signal degradation, and device longevity is crucial for ensuring the viability of invasive BCIs.
Future Directions: Expanding the BCI Horizon
- Non-invasive Advancements: Research is focusing on developing more sophisticated and user-friendly non-invasive BCI systems. Advancements in EEG technology, including dry electrodes, high-density arrays, and mobile brain imaging, hold promise for creating more portable, comfortable, and accurate non-invasive BCIs.
- Clinical Applications: BCIs are showing increasing promise for clinical applications, such as restoring lost motor function in individuals with paralysis, assisting in stroke rehabilitation, and treating neurological disorders like epilepsy and Parkinson's disease. Ongoing research and clinical trials are paving the way for wider adoption of BCIs in healthcare.
- Cognitive Enhancement: BCIs have the potential to enhance cognitive abilities, such as memory, attention, and learning. Research is exploring ways to use BCIs for cognitive training and to develop brain-computer interfaces that can augment human cognitive function.
- Brain-to-Brain Communication: One of the most futuristic and intriguing directions in BCI research is the possibility of direct brain-to-brain communication. Studies have already demonstrated the feasibility of transmitting simple signals between brains, opening up possibilities for collaborative problem-solving, enhanced empathy, and new forms of communication.
Resources for Further Learning and Development
- Brain-Computer Interface Wiki
- Research Journals and Conferences:some text
- Journal of Neural Engineering: https://iopscience.iop.org/journal/1741-2560 - A leading journal for BCI research and related fields.
- Brain-Computer Interfaces: https://www.tandfonline.com/toc/tbci20/current - A dedicated journal focusing on advances in BCI technology and applications.
Embracing the Transformative Power of BCI
From hybrid systems to adaptive algorithms, ethical considerations, and the exciting possibilities of the future, we've explored the cutting edge of BCI technology. This field is rapidly evolving, driven by advancements in neuroscience, engineering, and machine learning.
BCIs hold immense potential to revolutionize how we interact with technology, enhance human capabilities, restore lost function, and improve lives. As we continue to push the boundaries of mind-controlled technology, the future promises a world where our thoughts can seamlessly translate into actions, unlocking new possibilities for communication, control, and human potential.
As we wrap up this course with this final blog article, we hope that you gained an overview as well as practical expertise in the field of BCIs. Please feel free to reach out to us with feedback and areas of improvement. Thank you for reading along so far, and best wishes for further endeavors in your BCI journey!